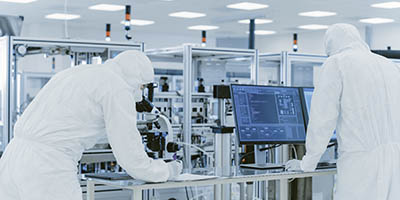
Knowledge Democratization with an Enterprise Knowledge Graph at Boehringer Ingelheim
Boehringer Ingelheim uses metaphactory to empower domain experts and deliver a seamless experience over interconnected, use case specific and use case agnostic knowledge graph applications.
The customer
Boehringer Ingelheim is working on breakthrough therapies that transform lives, today and for generations to come. As a leading research-driven biopharmaceutical company, the company creates value through innovation in areas of high unmet medical need. Founded in 1885 and family-owned ever since, Boehringer Ingelheim takes a long-term perspective. More than 52,000 employees serve over 130 markets in the three business areas, Human Pharma, Animal Health, and Biopharmaceutical Contract Manufacturing. In order to manage their constantly growing data and to gain new insights, Boehringer Ingelheim started the dataland program.
dataland – Main Goals
- Build a data-centric culture at Boehringer Ingelheim
- Implement technical systems needed to tackle data management challenges
The challenges
Constant data growth
- New data is generated by new studies, new research and new analyses
Siloed data handling
- Data is handled differently and within their own systems at each stage in the drug development pipeline
Limited data reuse
- Each use case generates new data without data reuse
The knowledge graph solution
Semantic model defined together with business
- The model captures domain-relevant concepts and relations
- Data references the semantic model directly
Explicit links with global identifiers
- Identifiers are globally unique and describe the data access protocol
Federation across data silos
- Multiple data silos and knowledge graphs can be accessed and interlinked using the SPARQL standard
Flexible and extensible data model
- The graph data model can be extended while improving data
- New data can be ingested before explicitly defining the semantic model
Data virtualization
- Tabular data outside of the knowledge graph can be mapped to the graph model
Seamless experience for domain experts
Collaborative modeling
- metaphactory's visual ontology editor allows non-IT users to describe their domain by capturing domain-specific concepts and relations in the model
- Data stewards, domain experts & business users at Boehringer Ingelheim actively contribute to the knowledge modeling process
Linked data exploration
- metaphactory enables end users to access and consume data across heterogeneous data silos without boundaries
- The Boehringer Ingelheim Linked Data Explorer powered by metaphactory provides interactive knowledge discovery and search interfaces that support use case specific interaction patterns
Example use cases
- Omics data management: Publishing of data from multiple laboratories to enable data scientists to reuse across business needs, use cases and analyses
- IT system management: Interlinking of data about tickets, incidents, IT systems, IT system leads and exploration of data in context
- Document management: Document exploration based on topic relevance
Key takeaways
- Domain experts and business users can learn to do modeling
An ontologist can support the modelling process at first, but non-IT experts should be empowered to contribute and extend the model to fit their needs - Embracing cultural change is critical
New roles have been defined to support the knowledge graph initiatives
- Data domain owners – users who own and are accountable for domain-specific data
- Data stewards – users who collaborate with IT to integrate data
- Use case agnostic applications allow data providers to focus on data creation and management
Learn more
To learn more about Boehringer Ingelheim's journey to building an enterprise knowledge graph serving multiple use cases and applications, have a look at this interview with Maksim Kolchin, Knowledge Graph Platform Lead, presented at the 2022 BioIT World Conference & Expo.
You can also read the interview in text format here »
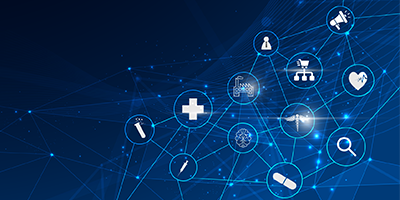
Enterprise Data Fabric at an American multinational biopharmaceutical company
As part of an enterprise-wide initiative to provide democratized, fluid, and connected data that drive business insights and decisions, this American multinational biopharmaceutical company uses metaphactory to deliver access to consumable and meaningful knowledge across systems and organizational boundaries.
By embracing a data-centric culture and adopting a semantic graph approach, the customer's enterprise data fabric promotes the re-use of data and drives convergence in the end-user analytics experience. Using this approach, end-user solutions are designed to use shared business-oriented data with well-defined meaning and linkages, while data is shared across individual applications.
This type of connected data fuels diverse insights that reveal dependencies between functions and processes - spanning the entire value chain from research and clinical trials, through production, to marketing and distribution - and is used as a basis for critical business decisions.
To learn more about this customer's journey to building a successful enterprise data fabric, have a look at this presentation by Dan Gschwend, Dir. Data Sciences, given at the 2021 Data-Centric Architecture Forum »

Knowledge hub for science & clinical analytics at an American multinational pharma corporation
Using metaphactory, this American Multinational Pharma Corporation built a science and clinical analytics knowledge hub that supports researchers, scientists and clinicians & bridges the gap between the research domain & the clinical domain.
The knowledge hub brings proprietary data (e.g., studies, experiments & other R&D entities) and public data (e.g., ChEMBL) together and provides domain users with a single entry point into the available data. The knowledge on-demand platform surfaces actionable and meaningful insights and makes knowledge accessible to and consumable by domain users. It helps accelerate R&D data discovery and understanding, and ultimately supports the team in bringing medicine to the market faster.
To learn more about this customer's journey to building a science and clinical analytics knowledge hub, have a look at this presentation by Sabine Schefzick, PhD, Director, Science and Clinical Analytics and Informatics, given at the 2021 Pistoia Alliance Spring Conference »
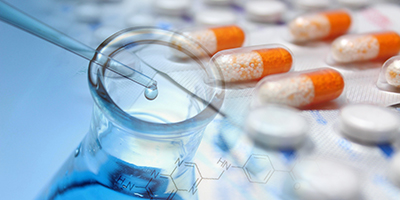
Drug development and drug repurposing at a Swiss multinational healthcare company
The target discovery application built with metaphactory on top of the customer's knowledge graph empowers data scientists, immunologists or systems biologists, to explore data and gain meaningful and actionable insights for their daily tasks.
The goal
Pharma companies have a lot of internal data about compounds they have developed and tested, clinical studies they have run, or research projects they are running. Whether these compounds, clinical studies or research projects have been successful or not, often the resulting data is difficult to access or reuse for new endeavours. On top of that, there is a considerable amount of public data available on compounds, drugs, diseases and their genetic associations, proteins and their coding genes, etc. Consequently, researchers struggle to analyze all this information and extract comprehensive insights from it.
metaphacts has joined forces with Ontotext to support this Swiss multinational healthcare company in building a knowledge graph based solution that provides highly interlinked information across various data sources and offers a modular approach to R&D data discovery and knowledge consumption.
One of the key use cases the customer focused on as part of their knowledge graph adoption and implementation journey was improving their current drug discovery process. The smart knowledge discovery solution they were building had the goal of helping researchers leverage all available proprietary and public data to:
- better use and repurpose existing drugs;
- find relevant targets for new drugs;
- perform targeted searches to find biomolecular information for specific indications or groups of indications.
The challenge
The main challenge was how to extract knowledge from data residing in multiple sources, in heterogeneous formats, and across various business units. In the existing drug development process, researchers looking to leverage preclinical or clinical data for particular compounds first had to find the relevant data sources. Then they had to search in each system independently, sometimes requiring further assistance from IT departments to perform their queries.
After collecting all required pieces of information, they had to integrate all different parts into a consistent report/record. This process was very time consuming and prone to errors, and even after all that effort, a lot of information still remained locked in unstructured data.
On top of that, as each of these reports/records related to one-off research, the resulting data, just like the data from previous drug testing (whether successful or not) was not reusable for other projects. Even when a new research had similar parameters to a previous project, researchers couldn’t build on existing results and had to start from scratch.
The solution: A preclinical knowledge discovery platform
The preclinical knowledge discovery platform jointly developed by metaphacts and Ontotext enabled the customer to transform and accelerate their drug development process. The solution covered the following steps:
- Modeling the domain with metaphactory, based on the Pharma company's specific information needs;
- Integrating all proprietary data with GraphDB and mapping the model to diverse data sources to build a customized knowledge graph;
- Providing access to relevant datasets from Ontotext's inventory of more than 200 preloaded public datasets and ontologies in RDF format, covering various knowledge domains (such as genomics, proteomics, metabolomics, molecular interactions and biological processes, pharmacology, clinical, medical and scientific publications);
- Building an intuitive user experience with metaphactory which allows end users to search and filter results, discover relevant information, bookmark and share results with their colleagues, add and edit data, build customized dashboards, etc.
The resulting application is rich and intuitive. It is also easy to adjust, extend and reuse to meet new business needs and cater to new use cases or end user groups. Thanks to its ability to represent data as a network of relationships, the created knowledge graph does not only provide access to diverse data sources, but also reveals previously unknown relationships in the data.
The solution employed a standardized data model, ontologies and vocabularies. Metadata was used to encode the meaning of the data and unique identifiers ensured that all meta-levels in the data were searchable, accessible, shareable and traceable. The resulting data makes it a lot easier to find, reproduce and reuse research results. It also includes clear provenance for addressing any data consistency issues coming from the highly dynamic environment of drug development.
Business benefits
- 5-6 weeks to go from idea to a production-ready solution;
- Adaptive data model to accommodate changing business needs;
- Leveraging proprietary knowledge with global data and keeping it up-to-date;
- Empowering end users to work with tons of diverse data and get meaningful insights;
- Driving digital transformation for better business outcomes.
Why choose metaphacts and Ontotext
With the new preclinical knowledge discovery platform developed by metaphacts and Ontotext, domain users at the customer site have fast and easy access to information via a live knowledge graph where the data for all integrated public datasets is constantly updated.
Combining a highly scalable and robust RDF database like GraphDB with a big inventory of ready-to-use biomedical datasets as well as Ontotext's proven methodology for semantic data integration enabled the customer to quickly create a large-scale customized knowledge graph.
On top of that, metaphactory's intuitive search and data exploration capabilities enable researchers working for this customer to interact with huge volumes of data consumed from the knowledge graph and use and reuse the knowledge locked in this data in a meaningful way.
Resources
To learn more about this solution, have a look at the customer talk Leveraging Knowledge Graphs for Drug Discovery given at the BioIT World Conference & Expo 2021 »